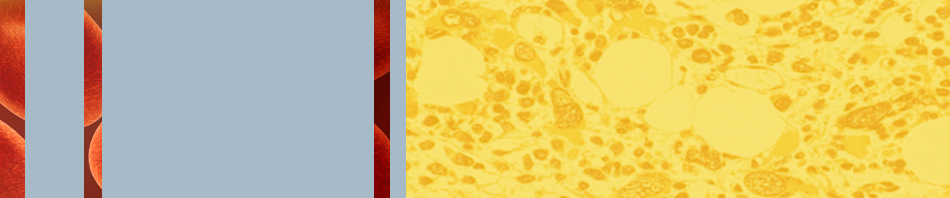
Researcher Profiles
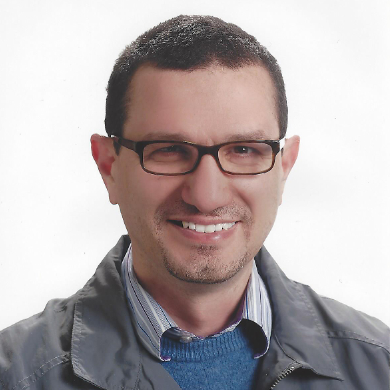
Issam El Naqa, Ph.D., F.A.A.P.M., F.I.E.E.E.
2023 Funding recipient
Use of machine learning to identify novel image-based markers to predict risk of developing MDS among patients with "pre-MDS" conditions.
Artificial Intelligence and Machine Learning in MDS 2023
PROJECT SUMMARY
Myelodysplastic syndromes (MDS) are a group of blood cancers where patients suffer from complications of low blood counts and often become in need for regular blood transfusions. The disease affects a patients’ quality of life and longevity. It is one of the most common types of blood cancer. MDS can transform overtime to acute myeloid leukemia. Over the past several years we learned more about the disease development where there is interplay between genetic mis- happenings (chromosome abnormalities or gene mutations) and inflammatory bone marrow environment which leads to ineffective production of blood cells. We know now that certain conditions pre-exist before development of MDS. Aging related clonal hematopoiesis is a phenomenon where we acquire genetic mis-happenings in some of our blood cells which can serve as the first hit of developing MDS. The diagnosis of MDS requires a highly specialized pathologist. There is significant discrepancy in making diagnosis of MDS between experienced pathologists in academic centers and community practices which can be as high as 40% and lead to 26% meaningful change in treatment or prognosis. There is also opportunity to prevent developing MDS if we conduct clinical trials among high risk “pre-MDS” conditions. Once MDS is diagnosed the most crucial step is risk assessment as treatment is tailored based on the disease risk and its chance to progress to acute myeloid leukemia. The modern risk stratification tools incorporate gene mis-happening data, blood counts and other clinical features. The molecular testing for MDS takes a long time to obtain and the costs are prohibitive in many countries worldwide.
We aim to take leverage of artificial intelligence (AI) tools to address some of the short comings described above: Who are the patients with “pre-MDS” conditions at highest risk of developing the disease? How can we assure right and accurate diagnosis? Can we improve and generalize risk assessment “staging the disease”?
We will develop digitized images from patients’ bone marrow aspirate and biopsy. We will use deep learning and machine learning (ML/DL) algorithms to interrogate those images. This will allow to examine so many salient features which can be used to predict developing MDS, assure diagnosis of MDS and aid in assessing the risk of the disease. Early data in small numbers of patients suggest that this approach is possible where better MDS assessment was provided. The AI analysis of morphologic features from bone marrow images predicted genetic mis-happenings and prognosis.
The study will be conducted on 600 patient’s microscopy images from the NHLBI MDS national study conducted by National Heart Lung and Blood Institute to develop the AI methodology and tools. Another 500 patients digitized tissue images from Moffitt Cancer Center will be used to validate those tools. We assembled team of experts in AI, MDS and pathology and in collaboration with NHLBI.
We hope that this work will translate to a widely available AI tool which can be utilized world-wide to improve prediction of developing MDS, aid in diagnosis and risk assessment using digital images of bone marrow aspirates and biopsies.